How Google’s “Deep Learning” Is Outsmarting Its Human Employees
mediatemple: How Google’s “Deep Learning” Is Outsmarting Its Human Employees: http://t.co/fIvUSpC2tJ via Fast Company *SC http://t.co/Q63I67AXUL
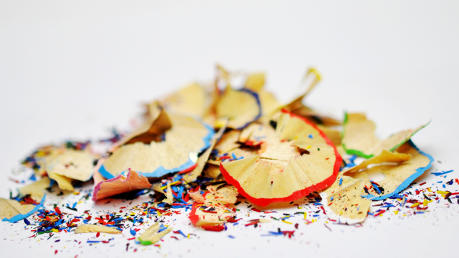
Google’s “deep learning” clusters of computers churn through massives chunks of data looking for patterns—and it seems they’ve gotten good at it. So good, in fact, that Google announced at the Machine Learning Conference in San Francisco that its deep learning clusters have learned to recognize objects on their own.
Traditionally, computers have been great at transporting data, but terrible at understanding what’s contained therein. The goal of movements like the semantic web have been to build webpages that understand what kind of content they’re serving, but advances have been slow in coming. Whereas common pigeons have the conceptual ability to tell the difference between a tree and a shrub, even the most expensive supercomputers today would struggle.
Google software engineer Quoc V. Le said at the conference that he realized the deep learning clusters had made a breakthrough when they were able to recognize discrete workplace objects, such as distinguishing two different brands of paper shredders. Interestingly, Lee didn’t train the machines this way—the software had figured that out on its own.
Le admitted that to program that level of classification, they’d have to reverse engineer the code from the results that the deep learning clusters spit out. “We had to rely on data to engineer the features for us, rather than engineer the features ourselves,” Quoc said during the talk.
To be clear, Google is not afraid that this will blow up into a fully sentient computer system—but it’s a stepping stone toward Google’s goal to get its computers solving menial problems in order to free up human engineers, who currently spend innumerable hours programming data processing solutions. Google’s using its deep learning clusters to better auto-recognize things in images, Android’s voice recognition, and Google Translate, among others.
Using the software framework DistBelief, Google harnesses tens of thousands of CPU cores operating under billions of parameters to run its deep learning clusters, a large-scale neural network that Google insists is nonetheless applicable to any gradient-based machine learning algorithm, regardless of size. In other words, let Google’s algorithmic deep learning model loose at any scale and it’ll learn to recognize things beyond your inefficient human ability.